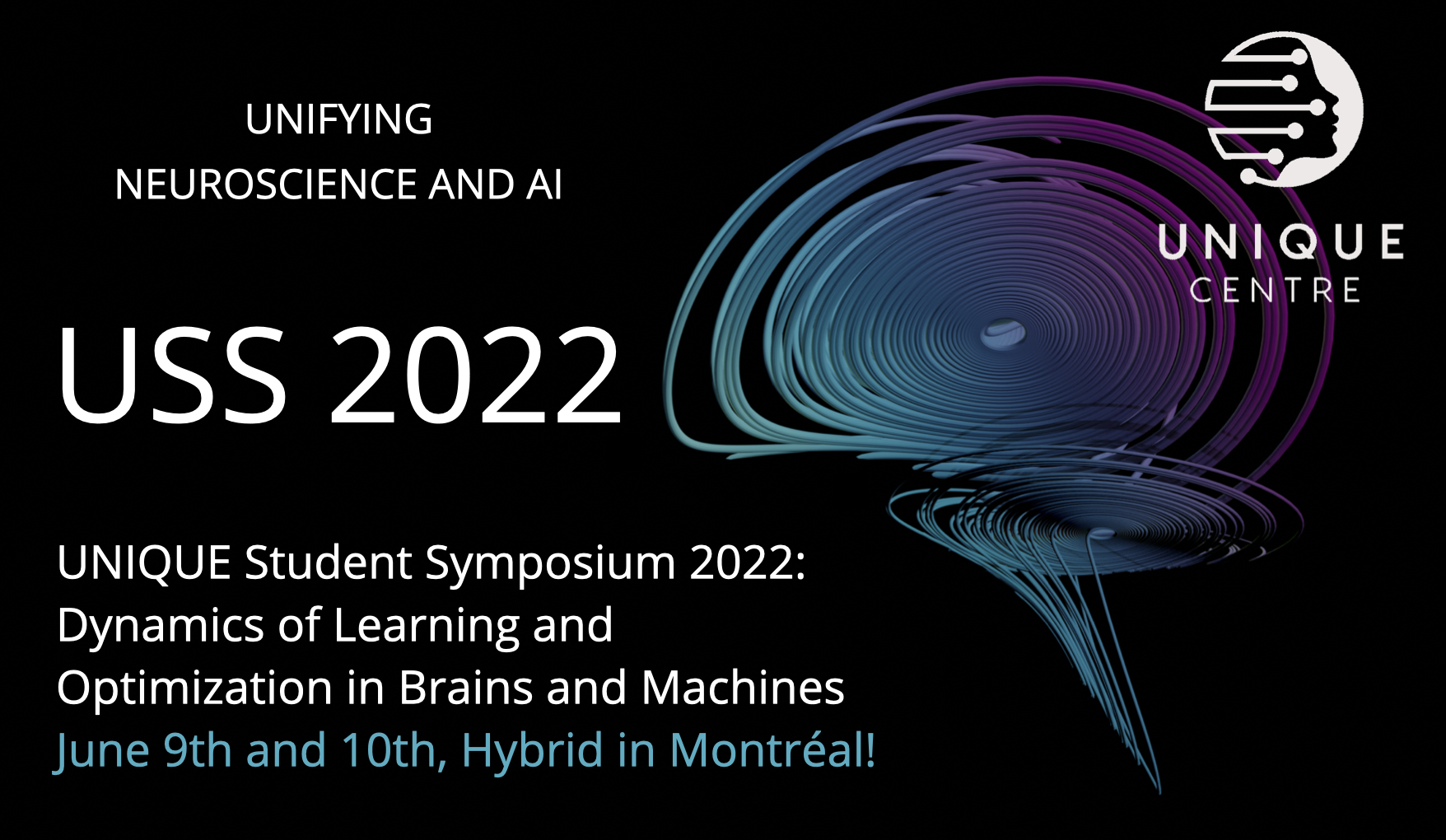
Accepted Posters and Lightning Talks
A Neurodynamic Model of Inter-Brain Coupling in the Gamma Band
Quentin Moreau, Lena Adel, Caitriona Douglas, Ghazaleh Ranjbaran, Guillaume Dumas
(Presented by Ghazaleh Ranjbaran)
The use of EEG to simultaneously record multiple brains (i.e., hyperscanning) during social interactions has led to the discovery of inter-brain coupling (IBC). IBC is defined as the neural synchronization between people and is considered to be a marker of social interaction. IBC has previously been observed across different frequency bands, including Theta [4-7 Hz]. Given the proximity of this frequency range with behavioral rhythms, models have been able to combine IBC in Theta with sensorimotor coordination patterns. Interestingly, empirical EEG-hyperscanning results also report the emergence of IBC in the Gamma range [>30 Hz]. Gamma oscillations’ fast and transient nature makes a direct link between Gamma-IBC and other (much slower) interpersonal dynamics difficult, leaving Gamma-IBC without a plausible model. However, at the intra-brain level, Gamma activity is coupled with the dynamics of lower frequencies through cross-frequency coupling (CFC). This paper provides a biophysical explanation for the emergence of Gamma inter-brain coupling using a Kuramoto model of four oscillators divided into two separate (brain) units. By modulating both the degree of inter-brain coupling in the Theta band (i.e., between-units coupling) and CFC (i.e., intra-unit Theta-Gamma coupling), we provide a theoretical explanation of the observed Gamma-IBC phenomenon in the EEG-hyperscanning literature.
A Spectral View of Recurrent Neural Networks Dynamics
Pingsheng Li, Jonathan Cornford, Arna Ghosh, Blake Richards
(Presented by Pingsheng Li)
Recurrent Neural Networks (RNNs) are powerful tools for both AI researchers and neuroscientists to understand neural activities and solve problems involving sequential, dynamical data and time series. Therefore, understanding how to better train RNNs becomes a pivotal question in AI and neuroscience. In this work, by employing tools from random matrix theory, we compare the effects of different initialization schemes in the performance of artificial recurrent neural networks, and perform a spectral analysis in order to understand and explain their differences in performance from the point of view of dynamical systems, which allows us to design a potentially better, spectrum-based hybrid initialization scheme for recurrent layers. Also, we perform the same analysis on two more biologically feasible RNNs, Song RNN and DANN RNN, which both incorporate Dale’s principal, i.e. a neuron can be either excitatory or inhibitory, but with different implementations. Our analysis shows that different implementations of Dale’s principal in RNNs can drastically impact the training and their computational capacities, which suggests that spectral analysis can be an effective method for analyzing both artificial neural networks and more biologically constrained neural networks as dynamical systems.
A comparative study of exploratory decision-making in mice, monkey, and humans
Veldon-James Laurie, Akram Shourkeshti, Cathy S. Chen, R. Becket Ebitz
(Presented by Veldon-James Laurie)
Every living species must have the capacity to make good decisions in a changing, uncertain world. However, gross differences in the behavioural adaptations across species mean we do not understand how our own ability to navigate uncertain environments evolved over time. Fortunately, there is a classic task we can use to compare decision-making across species within their own behavioural ecologies: the k-armed bandit. This task requires agents to choose between exploiting rewarding options and exploring options that can further develop their models of the environment. Here, we compared exploratory behaviours using this task across 3 species: mice, monkeys and humans. We found that mice responded significantly differently from monkeys and humans. Specifically, mice exploited less and explored more than both primate species. Analysis of the mice’s decision-making dynamics suggested that they had a reduced ability to persist in their goals. This comparative study highlights the differences in decision-making abilities between primates and mice and lays the groundwork for studies comparing neurodynamics in this task across species. Even though mice are more commonly used in preclinical neuroscience studies, this data suggests that monkeys may be a better choice for decision-making studies since they are more akin to humans in this domain.
A computational account of social interaction in children with psychiatric conditions
Lisane Moses, Guillaume Dumas, Baudouin Forgeot d'Arc
(Presented by Lisane Moses)
Autism Spectrum Disorder involves a variety of symptoms, with atypical social reciprocity being a primary one. Social reciprocity is an ambiguous concept that is measured in different ways by researchers, causing inconsistencies in findings on this topic. Nevertheless, we know that it is important to study this concept in the context of a dynamic exchange, which is not the case for all studies. An approach that places the subject in an active role would allow for a better categorization of the processes at play during social exchanges. An interesting approach is the penny hiding game: a player must guess in which hand his opponent has hidden a coin. A computational method, using mathematical equations to predict and/or describe behavior, has been used in previous research to determine the learning strategies used in autistic adults during the penny hiding game. The first goal of this project is to use this method to study learning strategies, but with children with autism. Then, it is relevant to ask if the atypical social functioning present in autism is also found in associated conditions. We want to compare the different conditions associated with autism in terms of social interactions. This project will allow us to better understand people with autism and their functioning in order to offer them appropriate interventions. It could also improve their quality of life by reducing the stress associated with social interaction.
Comparing Machine Learning and Inferential Statistics Approaches for the Classification of Cold Thermal Pain in Adolescents with and without Chronic Musculoskeletal Pain
Elizabeth Teel, Don Daniel Ocay, Stefanie Blain-Moraes, Catherine Ferland
(Presented by Elizabeth Teel)
Chronic pain is a leading cause of disability in adolescents, yet few objective methods of diagnosing pediatric pain exist. Our goal was to assess the potential of using EEG-derived features to detect thermal pain in adolescents with and without chronic musculoskeletal pain using machine learning (ML) and inferential statistics approaches. Thirty-nine healthy (15.2 +/- 2.1 years, 18 females) and 121 pain (15.0 +/- 2.0 years, 100 females) subjects had 19-channel EEG recorded at rest and during a cold-pressor task (CPT). Permutation entropy (PE), directed phase lag index, peak frequency, and graph theory features were derived along 10sec windows (Healthy: 292 rest & 273 CPT epochs; Pain: 1039 rest & 755 CPT epochs). Support vector machine (SVM) and logistic regression models classified between rest and CPT conditions. SVM models significantly distinguished between baseline and CPT conditions in pain (75.2% accuracy, 95%CI: 71.4–77.1%; p<0.001) and control (74.8% accuracy, 95%CI: 66.3–77.6%; p<0.001) subjects. Logistic regression models performed similar to the SVM (Pain: 75.8% accuracy, 95%CI: 69.5-76.6%, p<0.001; Controls: 72.0% accuracy, 95%CI: 64.5-78.5%, p<0.001). PE features in the theta frequency band were the largest contributors to model accuracy. Our results demonstrate the feasibility of accurately detecting subjective pain experience using EEG data, and represent the first step towards developing a point-of-care system to detect pain in the absence of self-report.
Comparing Optimal Decision-making Strategies
Alix Lavigne-Champagne, R. Becket Ebitz
(Presented by Alix Lavigne-Champagne)
There are many ways to make good decisions in changing environments. The two strategies most commonly studied are foraging and reinforcement learning (RL). RL comes from artificial intelligence (AI) development. Each possible action is associated with a reward value. However, the number of possible actions is often near infinite, which is costly computationally. Foraging, on the contrary, comes from the study of animal behaviour. Agents decide between exploiting one action and randomly exploring others. Because of this randomness, fewer calculations are required. The goal of this project is to find out which of these strategies leads to a higher reward. We compared the agents on k-armed bandits, a task where agents choose between 3 options whose rewards change unpredictably over time. Through simulations, we found the optimal parameters range for the two strategies in a set environment. The next step is to find the optimal strategy both for a rich and a poor environment. We hypothesize that foraging should yield better results in a rich environment as it is possible to access a lot of resources at random. Conversely, in a poor environment we hypothesize that reinforcement learning will be privileged because fewer resources are found at random. Through finding the environments where foraging is more optimal than RL, we will learn why foraging is so prevalent in animals and in what context(s) foraging could help AI development.
Comparison of face recognition in artificial and biological networks
Hamza Abdelhedi, Karim Jerbi
(Presented by Hamza Abdelhedi)
Computational neuroscientists have been investigating the similarity between artificial and biological vision for some time. Previous findings suggest that CNNs trained on object recognition capture the internal representations of the visual cortex. Nevertheless, as faces are a particular type of object and recruit specific regions in the brain, a stream of research has been investigating if CNNs also succeed in capturing the neural responses of the face-processing system. In this study, we address the question of similarity between the brain's internal representations and CNNs using MEG data acquired during presentation of 300 static face stimuli (Famous/Unfamiliar). We used 3 distinct architectures built for different purposes (FaceNet: Specifically built for Face Recognition, ResNet50: Built for Object recognition, and CORnet-S: Built to model the Visual Cortex). We found correlations between brain signals in visual areas and the activations in multiple artificial network layers. However, these correlations are not as strong as correlations observed in the task of object recognition. This suggests that CNNs trained on face recognition capture only a small part of brain activity patterns during face processing. Our results advance our understanding of the link between CNNs and neuromagnetic brain activity in face recognition tasks.
Distinguishing Learning Rules with Brain Machine Interfaces
Jacob Portes, Christian Schmid, James M. Murray
(Presented by Jacob Portes)
Despite extensive theoretical work on biologically plausible learning rules, it has been difficult to obtain clear evidence about whether and how such rules are implemented in the brain. We consider biologically plausible supervised- and reinforcement-learning rules and ask whether changes in network activity during learning can be used to distinguish which learning rule is being used. In particular, we note that supervised learning requires a credit-assignment model estimating the mapping from neural activity to behavior and that, in a biological organism, this model will inevitably be an imperfect approximation of the ideal mapping, leading to a bias in the direction of the weight updates relative to the true gradient. Reinforcement learning, on the other hand, requires no credit-assignment model and tends to make weight updates following the true gradient direction. We derive a metric to distinguish between learning rules by observing changes in the network activity during learning, given that the mapping from brain to behavior is known by the experimenter. Because brain-machine interface experiments allow for perfect knowledge of this mapping, we focus on modeling a cursor-control BMI task using recurrent neural networks, showing that learning rules can be distinguished in simulated experiments using only observations that a neuroscience experimenter would plausibly have access to.
Exploring cognitive profiles in healthy children using unsupervised machine learning
Myriam Sahraoui, Karim Jerbi, Bruno Gauthier
(Presented by Myriam Sahraoui)
The assessment of cognitive abilities in children is essentially made with neuropsychological tests. For example, design fluency tests are a great tool to assess executive functions, including planification and cognitive flexibility. Furthermore, artificial intelligence has increasingly became a common tool in the field of neuropsychology in the past decades, opening new avenues of research. Unfortunately, few studies have explored the use of artificial intelligence in the study of normal cognition in children. The aim of this study was to explore healthy children cognitive profiles based on the Five-point test (FPT), a design fluency test, with the help of unsupervised machine learning algorithms. The data of the FPT from 113 children aged 7 to 13 years old were collected on a numeric version of the test on tablet, and were used as input for two algorithms, namely the self-organizing maps (SOM) and the K-means. Unsupervised machine learning allows data driven exploration in children neuropsychology, a domain where those types of approach are still understudied. Preliminary results showed the cognitive profiles of the children were best separated into two clusters. And those clusters were separated based on the production of designs, number of repetitions and number of strategies used. Our study leveraged unsupervised machine learning to identify two cognitive profiles with distinct speed/accuracy tradeoffs during the performance of the task.
Fast streamlines search with Lpq Tree
Etienne St-Onge, Louis Collins
(Presented by Etienne St-Onge)
Fast Streamlines Search (FSS) is a novel method that can accurately and efficiently find all similar streamlines (k-nearest neighbors or radius search). This method can be used to cluster white matter bundles, trajectories, or connections estimated from diffusion MRI tractography. Compared to previous approximatives tractography search methods [O’Donnell 2007; Olivetti 2017; Garyfallidis 2018], FSS accurately computes the distance between every pair of streamlines streamline, within a given radius. To improve FSS computation speed, we implemented a k-d tree that internally uses the desired L2,1 distance. Furthermore, we generalized the Nanoflann k-d tree implementation [Blanco 2014] to any Lp,q Minkowski norm (1 ≤ p, q < ∞). When organizing the tree structure for proximity search, the proposed tree uses the absolute difference with a “scaling factor” to evaluate the boundary of each axis. This factor guarantees that there are no false dismissals (false negatives), based on “p”, “q” and the number of dimensions. When a sub-tree is located within that boundary it directly computes the exact distance, and returns similar streamlines accordingly. The open source implementation, named TractoSearch (https://github.com/StongeEtienne/tractosearch), could be further developed to incorporate advanced tractography segmentation or registration algorithms.
Loss of face identification abilities affect visual and semantic brain computations
Simon Faghel-Soubeyrand, Anne-Raphaelle Richoz, Delphine Waeber, Jessica Woodhams, Frédéric Gosselin, Roberto Caldara, Ian Charest
(Presented by Simon Faghel-Soubeyrand)
Here, we aimed to identify the specific neural computations underlying the loss of face identification ability by modelling the brain activity of brain-lesioned patient PS, a well-documented case of acquired prosopagnosia. We collected a large dataset of high-density electrophysiological recordings from PS and neurotypicals (N=19, total of ~65,000 trials) while they completed a one-back task on a succession of face, object, animal and scene images. PS showed archetypal behavioural deficits in this setting, with a larger behavioural impairment for face vs. non-face visual categories. We used Representational Similarity Analysis (RSA) to correlate human EEG representations with those of deep neural networks (DNN) models of vision and language-level semantics, offering a comprehensive characterisation of neural computations in PS and neurotypicals. EEG representational dissimilarity matrices (RDMs) were computed for each participant at 4 ms steps using cross-validated classifiers. PS’s RDMs showed significant within-subject reliability, indicating meaningful measurements of brain representations with RSA even in the presence of significant lesions. Using these RDMs, we found that PS’s brain had reduced neural distances between face-identity representations around 170 ms & 300 ms. Crucially, we found two neural signatures behind PS’s deficits: visual computations (corresponding to layers of a visual DNN) and high-level semantic computations (corresponding to language-level DNN).
The link between irrational action values and brain circular inference
Juvenal Bosulu, Yousra Mzireg, Sébastien Hétu
(Presented by Juvenal Bosulu)
Reinforcement learning (RL) models such as SARSA that compute expected values of choices are said to mimic the predictive functioning of the dopaminergic midbrain and striatum. Indeed, reward predictions are said to happen within the ventral striatum (VS) whereas reward prediction errors are computed within the dopaminergic (DA) ventral tegmentaL area (VTA). However, fewer work have looked into RL for irrational ‘wanting’, i.e. overestimation of reward that leads to maladaptive reward seeking, and is said to be related to sensitization of DA areas. Our hypothesis was that irrational ‘wanting’ and its multiplicative effect might be explained by the circular inference framework, which explains schizophrenia, hallucinations, etc. by an overcount of predictions and/or prediction errors through imbalance in inhibitory/excitatory mechanism that result in reverberation of predictions or prediction errors in a circular manner. We thus created SARSA agents in a normal versus in a circular grid world, in which the final reward could predict itself. We found that, in a circular environnement, the values of states that led to reward (and of the reward itself) were multiplied and much higher than the initial expected value of reward. Whereas, in a non circular environnement, the values of those states were never superior to the initial expected value, as it is normally the case in RL We show that circular inference combined with RL can explain some irrational forms of reward seeking.