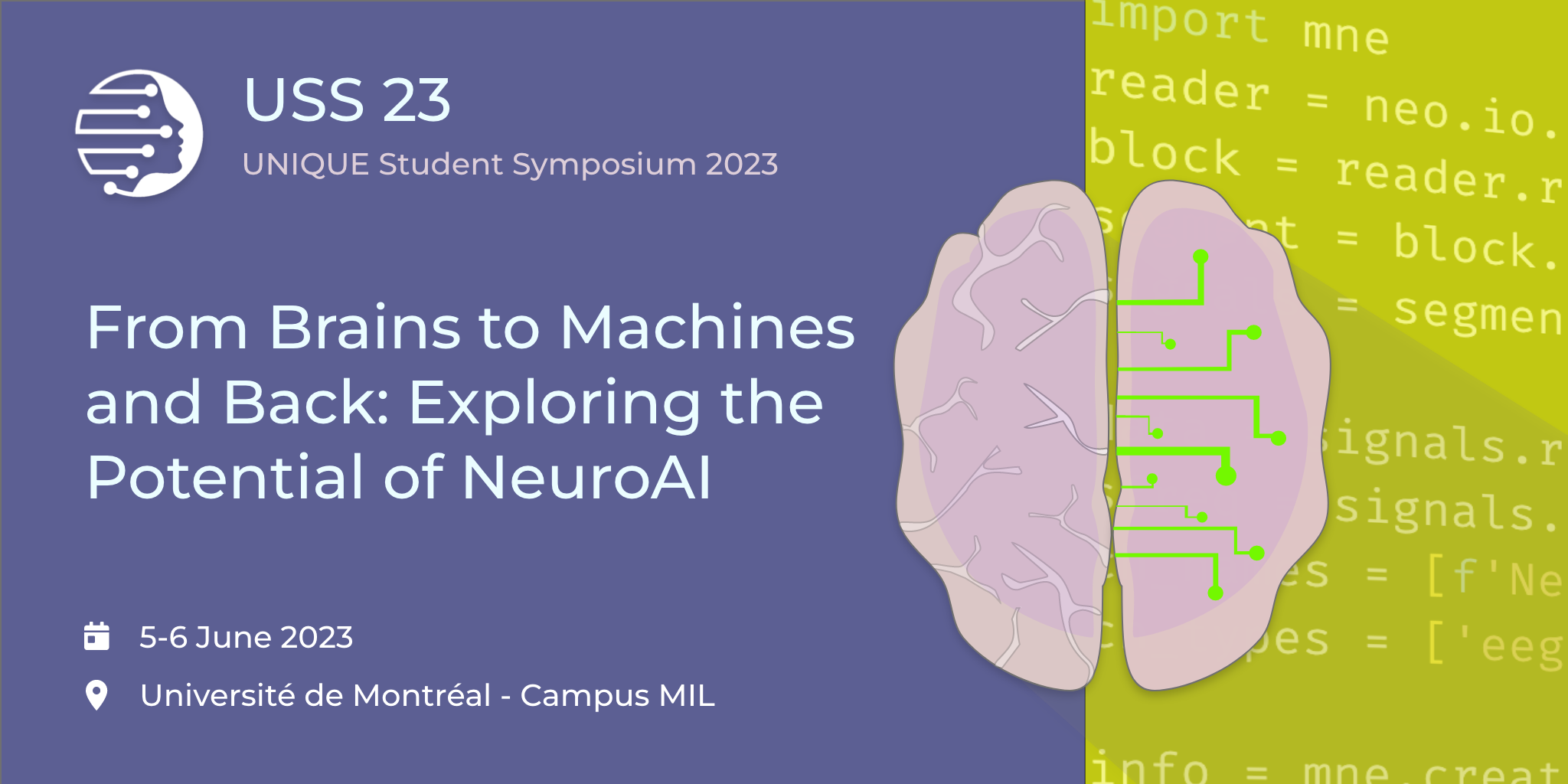
Accepted Posters and Lightning Talks
A deep learning framework for modeling and understanding visual perceptual learning
Maria Haddad*, Charlotte Volk*, Shahab Bakhtiari
(Presented by Maria Haddad and Charlotte Volk)
Poster
Training on a visual task results in long-lasting perceptual improvements, known as visual perceptual learning (VPL). Yet, VPL’s practical applications (e.g. rehabilitation) are limited as the benefits are often specific to the training stimuli. Developing a theoretical formulation of VPL can clarify our understanding of learning in the brain and improve its practicality beyond the laboratory. Recently, various works have demonstrated the potentials of deep learning and artificial neural networks (ANNs) in vision neuroscience. We are interested in extending this progress in two novel directions pertaining to VPL: (1) We use pretrained ANNs to generate stimuli that encourage the engagement of certain brain areas in a perceptual task. Previous works have shown that learning to engage higher visual areas during perceptual tasks leads to more generalizable training. Thus, we use “DorsalNet”, an ANN model of the dorsal visual stream, to generate stimuli that target higher dorsal visual areas during training. (2) We use deep learning as a theoretical framework to model perceptual learning at an algorithmic level. We investigate how various architectural components and learning rules can replicate the neuronal and behavioral features of VPL in ANNs. Our ongoing project, specifically, focuses on the role of skip connections in the dynamics and specificity of learning and their similarity with VPL. In this presentation, we will showcase our ongoing works along these two directions.
Accelerating Early Detection of Neurodegenerative Diseases Using Multimodal Imaging And Machine Learning
Olivier Valentin, Antonin Perrin, Alexandre Lehmann
(Presented by Olivier Valentin)
Poster and Lightning Talk
Alzheimer's disease is a prevalent neurodegenerative disorder affecting up to 6.5 millions of people in the United States. The disease is associated with faster rates of brain atrophy compared to healthy individuals, suggesting that Alzheimer's may be a form of accelerated brain aging. While current treatments for Alzheimer's only manage symptoms or slow disease progression, early diagnosis is critical for both patients and families to better understand the disease, plan for the future, and initiate therapies that may slow progression and improve management. Unfortunately, the current diagnostic methods for Alzheimer's rely on the documentation of significant cognitive decline, by which time severe brain damage has already occurred. Therefore, there is a critical need to develop a diagnostic tool that can detect early manifestations and accurately diagnose Alzheimer's in its earliest stages. Here, we present a machine learning approach able to predict participant’s age based on electroencephalography (EEG) data, with potential applications for developing a predictive tool to detect early premature aging of the brain. The best accuracy result was obtained using a Gradient Boosting classifier (76.6%, p<0.01) with a 0.5 learning rate. The correlation between the predicted and estimated age confirms the ability of our models to predict age. These results confirm that (1) aging affects human brain EEG signals, and (2) age can be predicted using resting state EEG data.
Changements physiologiques lors de la prise de décisions
Gabrielle Dufresne
(Presented by Gabrielle Dufresne)
Poster
Dans la vie quotidienne, les êtres humains sont confrontés à faire des choix. Certaines décisions ne nécessitent que peu de réflexion si elles ont déjà été prises dans le passé et se sont révélées comme étant un bon choix (exploitation). D’autres peuvent s’avérer plus complexes advenant le cas où cette option est nouvelle, ce qui signifie que les conséquences et les bénéfices du choix ne sont pas clairement établis (exploration). L’exploitation excessive peut donc être observée dans plusieurs conditions neurobiologiques, possiblement parce que l'exploration peut générer plus de stress que l'exploitation. Ce stress pourrait alors avoir un effet sur l'organisme, ce qui peut être mesuré par les changements observés dans le système nerveux sympathique. Toutefois, bien que cette hypothèse semble intuitive, elle n’a pas été testée empiriquement. Ainsi, l’objectif de cette étude sera d’investiguer les changements physiologiques sous-tendus par les stratégies d’exploration et d’exploitation. Les variations d’activité du système sympathique seront mesurées à partir d’un système de mesure physiologique et un modèle computationnel permettra de déterminer la stratégie utilisée: exploration ou exploitation. Cette étude contribuera à une meilleure compréhension physiologique de la raison pour laquelle certaines décisions semblent plus stressantes que d’autres et pourrait éventuellement permettre de créer des modèles de prise de décision qui imitent le comportement humain.
Characterizing the behavioral signatures of the marmoset model through DeepLabCut, a deep learning program
Jiayue Yang, Dominique Bédard, Philippe Huot, Justine Cléry
(Presented by Jiayue Yang)
Poster
As a small primate, the common marmoset has been proposed as an ideal preclinical model for human diseases, such as Parkinson, to bridge the gap between rodent research and clinical trials. As changes in the behavior can reflect early biomarkers of disease onset and progression, our goal is to assess and quantify the behavior in healthy animals first. For this, we are using DeepLabCut, a deep learning program for noninvasive animal behavior tracking in laboratory uses. In our lab setting, we use cameras in the animals’ cages to record their daily activity and movements, then we use DeepLabCut to build a skeleton with spatially labeled body parts to detect the typical behavioral components of marmosets. This project represents the first step of marmoset behavioral analysis to develop diagnostic and prognostic biomarkers for Parkinson’s Disease, which can be eventually translated into use in human diagnosis and future clinical care. In the context of Parkinson’s disease, one specific relevant priority is to use computational tools that aid in the identification of motor and cognitive deficits. With the use of the marmoset model, my project with contribute to this priority by providing more accessible and efficient measurements for behavioral dynamics within animal infrastructures. Therefore, the proposed project will promote the use of computational models in animal models for behavioral analysis and further applications of relevant research projects in our lab.
Choosing wisely: Exploring Decision-Making Differences in Mice, Monkeys, and Humans for Advancing Artificial Intelligence
Veldon-James Laurie, Akram Shourkeshti, Cathy S. Chen, R. Becket Ebitz
(Presented by Veldon-James Laurie)
Lightning Talk
One of the goals of AI is to attain human-level intelligence. When aspiring for these advances, we often turn towards neuroscience, where data is more often collected from other species, such as mice and monkeys. However, gross differences exist across species because of behavioral adaptations, making it difficult to determine how these other species compare to human intelligence. Fortunately, to compare these species we can use a classic task: the k-armed bandit. This task requires agents to choose between exploiting rewarding options and exploring options that can further develop their models of the environment by gaining information about the rewards of other options. Here, we compared exploratory behaviours across 3 species: mice, monkeys and humans. We found that mice behaved significantly differently from monkeys and humans. Specifically, mice exploited less and explored more than both primate species. Analyses of the mice’s decision-making dynamics suggested that they had a reduced ability to persist in choosing the same option repeatedly, compared to the primates. This comparative study suggests that training AI models using non-human primate data, compared to mice data, may lead to more human-like decision-making abilities in AI systems. Overall, this study highlights the importance of considering differences in decision-making strategies across species when developing AI models that aim to emulate human intelligence.
Cognitive control over reflexive saccades
Rishabh Singhal, Devin Kehoe, R. Becket Ebitz
(Presented by Rishabh Singhal)
Poster
A saccade is the intentional movement of the eye from one target to another. Saccade latency distributions reveal distinct groups of saccades, including a group of visually-guided reflexive eye movements, referred to as express saccades. We still do not know how fundamental cognitive variables, like reward and goal states, play a role in expressing saccades. In this study, rhesus macaque performed a classic decision-making task known as multi-arm bandit. At the trial beginning, the subjects fixated their eyes on a central spot, following which three targets would appear on the screen. Then the subjects chose one of the three targets by saccading to it. Although the targets appeared visually identical, each had a different reward rate, changing with time. In an uncertain environment, one does not always know the best option. Hence, one must explore other targets that might be better, disregarding immediate rewards. We used a hidden Markov model to identify latent explore and exploit goals. We analyzed the effect of reward history on express saccades during the task. The reward history did indeed influence the probability of generating express saccades. Subsequently, we compared the response time during explore vs. exploit goal states. The goal state effects on express saccade probability appeared larger than the effects of reward history. These findings indicate that express saccades may be linked to decision-making variables in the brain, like reward history and goal states.
Comparing Decision-Making Algorithms using Recurrent Neural Network
Paul-André Robillard, Alex Chang, Alix Lavigne-Champagne, R. Becket Ebitz
(Presented by Paul-André Robillard)
Poster
When we have multiple options in front of us, we all want to make the best decision. Different scientific domains present different algorithms as the optimal strategies, but directly comparing these algorithms remains difficult. Here, we use recurrent neural networks (RNN), to compare two influential decision making algorithms: reinforcement learning (RL) and foraging. The RL algorithm compares the values of each available option and selects the option with the highest value. The foraging algorithm, however, compares a single option against some threshold and ignores the other options. We hypothesized that the brain would use the foraging algorithm because it would be more efficient to keep track of one value than all values. In a task where the algorithms make one choice out of 3 options, we used imitation learning to train recurrent neural networks to mimic the behavior of the RL and foraging algorithms. We performed principal component analysis (PCA) on the RNN which revealed similar dimensionality for RL and foraging indicating similar computational demands, refuting our initial hypothesis that foraging is less computationally demanding. However, the 2D and 3D representational graphs showed a string-like (one string for each choice) representation of choice for foraging versus a more evenly distributed representation for RL. Our next step will be to compare RNN trained on human behavioral data to see if the structure and representation are more apparent to RL or foraging.
Computational phenotype during social interaction of children with psychiatry conditions
Katelyn Cassar, Quentin Dufranne, Pauline Ligonie, Theo Badra, Baudouin Forgeot d'Arc, Guillaume Dumas, Lisane Moses
(Presented by Katelyn Cassar)
Poster
Autism spectrum disorder (ASD) is a heterogenous condition involving symptoms of atypical behavior in social reciprocity due to a presumed deficit in Theory of Mind (ToM). This criterion allows differential diagnosis from other psychiatric conditions that are also highly comorbid in ASD such as attention deficit – with or without – hyperactivity disorder (ADHD) and anxiety disorder (AD). However, ToM measures are usually not objective and poorly ecological. Here, we want to study the computational phenotype associated with ToM (sophistication, flexibility, and alternance/perseveration) and the adaptation strategy profile in ASD (n = 28), ADHD (n = 34), AD (n = 34) and neurotypical (NT; n = 30) pediatric populations using a quantitative ecological task. Using a Variational Bayesian approach, we extracted individual computational phenotype and established their adaptation strategy profiles. Results showed group differences in performance, no significant effect of sophistication nor flexibility, more perseveration in ASD than in other groups, and that most subjects used a reinforcement learning strategy. Performance and alternance/perseveration were also associated with questionnaires measuring the symptoms of the studied conditions. These results highlight the importance of studying children with psychiatric disorders since their behavioral phenotype differs from adults and that diagnostic criteria based on mentalizing deficits might not be well suited for children.
Cross-modal few-shots learning
Antoine Bou Khalil, Sylvain Baillet
(Presented by Antoine Bou Khalil)
Poster
Fast-mapping (FM), a learning paradigm in which new knowledge is acquired incidentally through inferences, has been widely investigated in the past few years, both at the behavioural and neural levels. Its characteristics make it a model of choice for developing fast learning algorithms: studies have shown that it may allow direct neocortical few-shot encoding on a reduced time scale, thus differing from the well-known mechanisms of explicit encoding (EE), which often require hundreds of learning events. However, one limit of FM is its greater sensitivity to interferences possibly caused by the high uncertainty associated with inferential learning. Although both FM and EE have been compared for their resistance to interferences, to the best of our knowledge, the effects of combining the two learning methods have never been investigated. Given that higher uncertainty leads to higher learning rates, we hypothesize that cross-modal learning may outperform single-modal learning. In this study project, we propose to assess knowledge primarily acquired with FM by following it up with confirmative or contradictive EE and reversely, on the same-day or after a night’s sleep. This extensive behavioural task combined with the recording of the event-related potentials elicited by the second informative event would help us characterise the uncertainty associated with fast-mapped knowledge, thus giving us an insight into creating more rapid yet robust machine-learning algorithms.
De-localising the training loss in AI models for tractography
Emmanuelle Renauld, Antoine Théberge, Pierre-Marc Jodoin, Maxime Descoteaux
(Presented by Emmanuelle Renauld)
Poster
Many tasks using MRI have been the object of machine learning (ML) studies, but tractography is probably the most challenging. There is a duality in tractography: it requires the local direction to be aligned on the diffusion signal, but also the overall shape of the tracks to represent real fiber pathways, connecting adequate cortical regions. This led to two main types of algorithms: global vs iterative. When using the latter, which only uses local information, tweaks are necessary to include anatomical priors, although it is still unclear how to do that. One hope of ML was that it could be a way to include those priors, particularly using sequence-based algorithms such as RNNs, which have a «memory» of the provenance of the streamline. But their loss function is still local, providing no insurance that the final shape will be good. The hyperparameter research is thus complicated: developers need to verify metrics on a whole generated tractogram. Here, we suggest that not all points of the streamline have the same importance. Points where the streamline is smooth and straight are easy to track and do not provide insights on the general path of the streamline. Because of the exposure bias, a very poor algorithm could learn only to copy the previous direction and still get a near-perfect loss. Points where the streamline changes direction should have more weight in the final loss. We propose a compressed loss and show preliminary results.
Fingerprints are forever: healthy aging alters the content of neurophysiological fingerprints but not their accuracy
Jason da Silva Castanheira, Alex Wiesman, Sylvain Baillet
(Presented by Jason da Silva Castanheira)
Poster and Lightning Talk
Brain fingerprinting is quickly changing our neuroscientific toolkit for understanding inter-individual diversity in brain activity. In our present paper, we used magnetoencephalography (MEG) to explore neurophysiological brain fingerprinting throughout healthy ageing. We derived anatomically specific neurophysiological fingerprints of 606 individuals ranging from 18 years old to 89 based on frequency-defined spectral features of eight-minute recordings. We achieve high individual differentiation accuracies (~90%) for the entire cohort and across age groups (18-45 years old, 92%; 65-89 years old, 93%). The most salient neurophysiological features for differentiating all individuals predict individual differences in performance on tests of fluid intelligence. The most differentiable neurophysiological features within age groups change along a superior-to-inferior gradient. Older adults are differentiated from one another using brain activity in superior unimodal cortices. These same brain regions are where we best decode general cognitive abilities and demonstrate the greatest age-related cortical thinning. Our study showcases the robustness of neurophysiological brain-fingerprinting throughout aging and emphasizes the importance of considering divergence in the underlying contents of brain-fingerprints when studying individual differences in populations with varying demographics and cognitive abilities.
Flexible Phase Dynamics for Bio-plausible Contrastive Learning
Ezekiel Williams, Colin Bredenberg, Guillaume Lajoie
(Presented by Ezekiel Williams)
Poster and Lightning Talk
Many learning algorithms used as models in neuroscience or as candidate approaches for learning on neuromorphic chips learn by contrasting one set of network states with another. These Contrastive Learning (CL) algorithms are traditionally implemented with temporally non-local, and periodic learning dynamics that could limit the range of physical systems capable of harnessing CL. In this study, we build on recent work exploring how CL might be implemented by biological or neurmorphic systems and show that this form of learning can be made temporally local, and can still function even if many of the dynamical requirements of standard training procedures are relaxed. Thanks to a set of general theorems corroborated by numerical experiments across several CL models, our results provide theoretical foundations for the study and development of CL methods for biological and neuromorphic neural networks.
Hierarchical modularity optimizes memory capacity near criticality in echo state networks
Filip Milisav, Andrea Luppi, Laura Suárez, Bratislav Misic
(Presented by Filip Milisav)
Poster and Lightning Talk
The complex architecture of brain networks is believed to be organized in a hierarchy of increasingly polyfunctional nested circuits. Previous reports have suggested that this hierarchical modular structure contributes to maintain a balance between information segregation in specialized neuronal communities and global integration via intermodular communication. Yet, how hierarchical modularity shapes network function remains unclear. In this study, we constrain echo state networks with wiring patterns inspired by the hierarchical modular topology of biological neural networks. This allows us to causally relate hierarchical network architectures to cognitive performance in a simulated memory task. Furthermore, we characterize the computational capacity of these neuromorphic networks across a range of dynamics, bridging stable and chaotic regimes. We find that higher-order hierarchical modularity optimizes memory capacity in echo state networks. The impact of such a nested modular organization reaches its peak at criticality – a dynamical regime at the “edge of chaos”, which might explain recent results showing that echo state networks constrained with human brain connectivity patterns perform optimally near criticality. Here, this boost in performance is achieved by tuning intermodular connectivity, possibly modulating permeability of information flow between functionally segregated communities in a way that strikes a balance between information segregation and integration.
Impact of Initialization Techniques on the Performance of Deep Learning Approaches for Pediatric Intra-Subject MRI Registration
Andjela Dimitrijevic, Benjamin De Leener, Vincent Noblet
(Presented by Andjela Dimitrijevic)
Poster
Deformable image registration aligns two images into a common reference frame for normalized visualization between brain scans. However, current methods are less adapted to pediatric data, leading to less accurate registration due to bigger volume differences when analyzing longitudinal data with larger age intervals. To improve registration tasks in the pediatric context, researchers have turned to deep learning (DL) based techniques, which can estimate deformation fields directly from pairs of 3D images, making it a quicker alternative to traditional methods. To address this, we evaluated three unsupervised DL-based approaches for intra-subject deformable registration, including with and without pre-initializations. Our results showed that the DL-based approach achieved similar results to the conventional SyN ANTs method, and the registration quality in terms of tissue segmentation overlap using the Dice score was significantly higher for approaches with pre-initializations. The rigid and affine pre-initialization approach demonstrated the smallest percentages of negative Jacobian determinant values and statistically significant higher Dice scores than SyN ANTs for white matter and gray matter segmented regions. This study presents a promising approach to improving the accuracy and efficiency of intra-subject registration in pediatric longitudinal brain imaging, which has important implications for the creation of diagnostic pipelines using individual to template matching.
Multi-brain Decoding for Precision Psychiatry
Ghazaleh Ranjbaran, Guillaume Dumas
(Presented by Ghazaleh Ranjbaran)
Poster
Autism spectrum disorder (ASD) is a neurodevelopmental disorder that is primarily characterized by atypical social interactions. Traditionally, social cognition in individuals with ASD has been studied by analyzing brain signals of single participants in isolation. However, in recent years, hyperscanning techniques have been developed, which allow the recording of brain signals from multiple individuals engaged in social interactions. This has opened up new possibilities for studying inter-brain dynamics and identifying physiological markers of ASD. In this project, the Banville et al. self-supervised learning tasks for EEG data will be adapted to improve downstream classification performance. Specifically, shallow and deep FBCSNet models that consist of temporal and spatial convolution units with a linear layer will be employed for the classification task. These models will classify single-brain EEG data for four motor imagery tasks, and will then be used in a multi-brain architecture for hyperscanning-EEG classification. By leveraging single-brain convolutional neural networks in the multi-brain architecture with SSL algorithms, a predictive model will be fit at the individual level with limited data samples. This is a crucial step towards the efficient combination of computational models and neurotechnology in precision psychiatry.
Neuromodulation of the Right Temporo-Parietal Junction in Human-Machine Social Interactions
Vincent Chamberland, Lisane Moses, Guillaume Dumas, Quentin Moreau
(Presented by Vincent Chamberland)
Poster
The neurophysiological processes underlying social interactions remain largely unexplored in neurophysiology, primarily due to the challenges of designing experimental protocols involving multiple participants. However, the recent introduction of virtual partners (VPs) allows for the recreation of realistic social situations while maintaining a high level of experimental control. The Human Dynamic Clamp (HDC) is an experimental paradigm that measures the degree of social interaction between a naive participant and an adaptive VP during a coordination task. Studies that combined HDC and electroencephalography (EEG), a functional neuroimaging technique, have identified the right temporo-parietal junction (rTPJ) as a significant neuronal correlate of our ability to interact with other agents, albeit without establishing causality. This research aims to combine HDC, EEG, and transcranial random noise stimulation (tRNS), a neuromodulation technique, to investigate the causal role of rTPJ in our social interaction abilities. Preliminary results hint towards an improvement in synchrony scores and humanness attribution following online stimulation of rTPJ during the social interaction task with the VP.
Normative modelling on EEG data distinguishes neurodevelopment disorders from neurotypical population
Adrien Dubois, Guillaume Dumas, Sébastien Jacquemont
(Presented by Adrien Dubois)
Poster
Neurodevelopmental disorders (NDDs) present a variety of symptoms and etiologies, which poses challenges for accurate diagnosis and personalized treatment. Although EEG has demonstrated potential in revealing the neural basis of NDDs, inconsistent findings and methodological heterogeneity have impeded its practical applications. Furthermore, reproducibility and interpretability remain critical concerns in medical research. Our study aims to overcome these challenges by creating comprehensive models and integrating multiple databases into a single framework. We hypothesize that an enhanced approach to electrophysiology can identify reliable biomarkers of NDDs, enhance biological interpretability, and advance the development of actionable biomarkers and better understanding of biological mechanisms. We established standardized growth charts of electrophysiological measures using normative modeling on a large-scale dataset (N=5000) obtained from diverse cohorts. This approach accounts for nonlinear age-related effects on EEG measures, gender and sex variations, and different cohorts, enabling rigorous statistical analyses of large-scale multi-site data. We found significative differences of power in each frequency bands between neurotypical and ASD. Future studies will aim at explaining the physiology behind those differences and integrate cohorts from others NDD.
Scaling Multi-Brain Computational Neuroscience with Networks of Coupled Oscillators: from Biological Mechanisms to Technological Measurement
Lena Adel, Quentin Moreau, Ghazaleh Ranjbaran, Guillaume Dumas
(Presented by Lena Adel)
Poster
Hyperscanning allows recording multiple brains during interaction to measure inter-brain synchronization (IBS), which is linked to social behaviors. However, some aspects of IBS are not yet fully understood. Few studies have investigated neural dynamics at the inter-brain level using simulations, partly due to the complexity of such simulations. In this study, we use a small number of oscillators to address two controversies encountered in EEG hyperscanning: the mismatch between time-scales of IBS in Gamma oscillations and information communicated during social behavior, and the lack of guidelines on robustness and sensitivity of measures for detecting IBS. We simulate neural oscillations in two interacting brains using the Kuramoto model. We use our open source pipeline (HyPyP) for inter-brain connectivity analysis and assess its validity and sensitivity to biophysical and technical factors. Our simple biophysical model, using 4 oscillators, successfully explains the emergence of IBS in the gamma band through cross-frequency coupling. A second model using 6 oscillators shows the limitations of small networks to exhibit proper complex dynamics. More complex simulations are required to benchmark EEG inter-brain connectivity measures. Computational models offer meaningful insights for multi-brain neuroscience. Simple models can test hypotheses about specific biological mechanisms underlying IBS, but larger models are required to investigate complex dynamics involving delays.